In the early 20th century, the primary purpose for mental disorder classification was to organize asylums.{[1] The process for categorizing symptoms lacked standardization and thus was inconsistent. Even so, the categories were preserved so psychiatrists could collect statistics for public policy and funding. After time progressed and techniques became more sophisticated, the American Psychiatric Association created the Diagnostic and Statistical Manual of Mental Disorders (DSM) in 1952. Serving as a guide to diagnosis, the primary purpose of the DSM was to standardize the classification of mental disorders. The first edition of the DSM contained nearly 100 disorders that were characterized by behavioral symptoms. Over the past 60 years, the DSM has been the standard for diagnosing mental disorders. [1] Although the most recent edition of the DSM, the DSM-V, has improved specificity and consistency in its criteria, it still holds the potential for inaccurate diagnosis. [2, 3] With the exception of drug dependency, the DSM predominantly considers emotional and behavioral symptoms rather than biological symptoms. [4]
In the early 20th century, the primary purpose for mental disorder classification was to organize asylums [1]. The process for categorizing symptoms lacked standardization, and thus was inconsistent. Even so, the categories were preserved so psychiatrists could collect statistics for public policy and funding. After time progressed and techniques became more sophisticated, the American Psychiatric Association created the Diagnostic and Statistical Manual of Mental Disorders (DSM) in 1952. Serving as a guide to diagnosis, the primary purpose of the DSM was to standardize the classification of mental disorders. The first edition of the DSM contained nearly 100 disorders that were characterized by behavioral symptoms. Over the past 60 years, the DSM has been the standard for diagnosing mental disorders [1]. Although the most recent edition of the DSM, the DSM-V, has improved specificity and consistency in its criteria, it still holds the potential for inaccurate diagnosis [2][3]. With the exception of drug dependency, the DSM predominantly considers emotional and behavioral symptoms rather than biological symptoms [4].
Psychiatrists in the early 1900s simply did not have the ability to determine the biological causes of mental illness, but today, developing technologies have the potential to do so. For instance, genome-wide association studies (GWAS) examine the human genome in an attempt to link the gene loci, locations of genes and mutations on a chromosome, to psychiatric symptoms [5]. GWAS attempts to correlate the frequency of genetic markers with the frequency of symptoms [5]. At their creation in the early 2000s, GWAS were thought to be the solution for linking genetic loci to diseases or mental illnesses [6]. It turns out that these studies are useful for determining candidate genes that may be linked to a mental illness. However, the main downfall for GWAS is that mental illnesses are not linked to a single genetic locus, but rather a combination of loci [2][5]. With around 25,000 protein-coding genes in the human genome to consider, it is extremely difficult to determine the combinations of contributing genetic loci [5].
The emerging field of computational psychiatry provides the possibility of deducing the relationships between the physiological and emotional symptoms of mental illness, with the goal of eventually integrating these findings into diagnostics and treatment. Computational psychiatry utilizes machine learning, a process in which algorithms comb through large amounts of data to discover and predict patterns, to predict the correlation between biological markers and behavioral symptoms of mental illnesses [5]. When machine learning is applied to psychiatry, it enriches the field because of its generative model, which is unlike an update of the DSM [3]. In a generative model, algorithms use probability and statistics to determine what causes will generate a certain result, such as a specific mental illness. One statistical method used is Bayesian inference, which is when an algorithm updates the probability of a hypothesis as it analyzes more and more data. After many updates of the hypothesis, the probability of that hypothesis becomes more accurate. To put this in perspective, the Schizophrenia Working Group of the Psychiatric Genomics Consortium used algorithms to test 9.5 million combinations of genetic loci before drawing a conclusion about gene expression and schizophrenia [7]. Luckily, as medical records and research become more accessible due to increased use of digital storage, there are many opportunities to begin implementing these algorithms and better understand how our biology influences behavior [5].
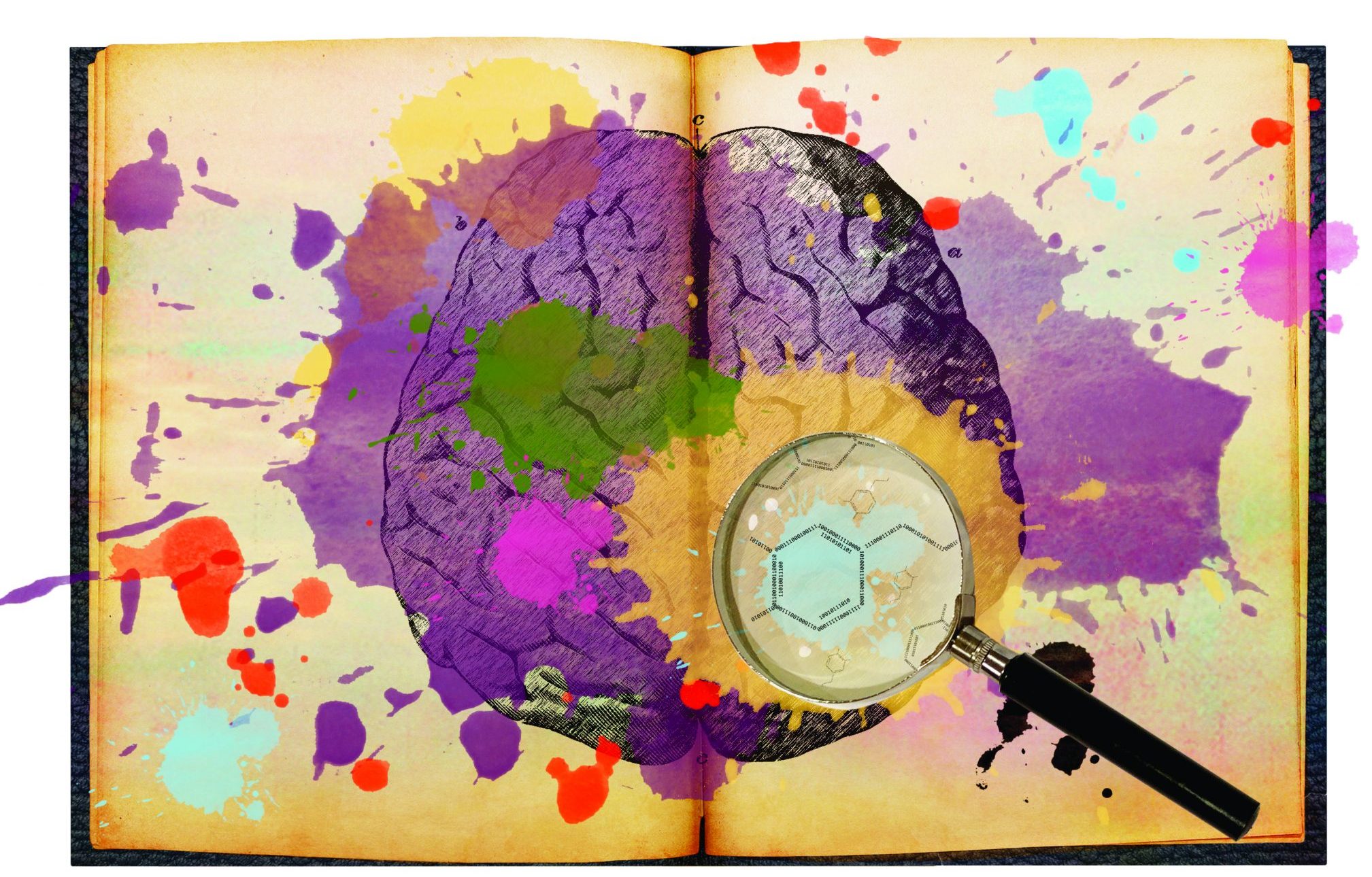
In fact, through various competitions and research, breakthroughs have already taken place for integrating computational psychiatry into schizophrenia diagnosis. The annual Machine Learning for Signal Processing (MLSP) competition, which has been running for ten years, attempts to test the current restrictions of automatic diagnosis for schizophrenia patients. The results of the competition improve from year to year, as contestants are able to use more data and learn from previous techniques. The 2014 competition had contestants use MRI scans to try and predict the patients with schizophrenia with the greatest accuracy [8]. MRI scans capture images of the human brain, allowing doctors to view the condition and functioning of an individual’s nervous system in various manners. More specifically, a functional MRI (fMRI) uses blood oxygen level-dependent signals to assess the brain’s functional network connectivity, which suggests how the neurons are interacting in the brain. Previous research has suggested certain neural interaction patterns with schizophrenia, making fMRIs a useful tool for collecting neural interaction data that can then be translated into diagnostic data by machine learning. Similarly, structural MRI (sMRI) scans, which determine the density of gray matter, white matter, and cerebrospinal fluid in the brain, may also be linked to schizophrenia diagnosis. The winning team created a Gaussian process, a multivariable normal distribution of random variables, of large scale data. The research from the MLSP competition demonstrates that when data from fMRIs and sMRIs are analyzed by algorithms, a complete picture of brain organization can be described and predict the causes for mental illnesses, likely improving future diagnosis and treatment [8].
Applying computational psychiatry to schizophrenia has useful implications for psychiatry as a whole. The goal of computational psychiatry is to paint a fuller picture of mental illness, allowing psychiatrists to effectively diagnose their patients through both abstract perspectives of emotional symptoms and concrete aspects of computational data. For example, one complication with diagnosing schizophrenia is that it is often diagnosed through an elimination process that first considers other options such as bipolar disorder [8]. It has been suggested that schizophrenia has similar symptoms to bipolar disorder in terms of thalamo-cortical circuit dysfunction, but is distinct when it comes to the variance of cortical activity [2]. Algorithms, such as those created in the MLSP competition, aim to reduce misdiagnosis by creating a more automatic diagnosis [8]. Computational psychiatry can take both emotional and physiological symptoms into account effectively determining the separation between the overlap and identifying symptoms unique to bipolar disorder and schizophrenia [2]. This process could be extended to many other mental illnesses, creating a list of symptoms that considers both the emotional signs and biological markers of the diagnosis. Furthermore, a study from 2014 combined data from multiple GWAS and discovered that the dopamine receptor 2 was one of the notable gene loci for schizophrenia, which is significant because it is successfully targeted by antipsychotic drugs [7]. Using machine learning to analyze large amounts of GWAS data may yield results more accurate than a single GWAS test on its own. If machine learning can determine the necessary target loci for medication for other mental illnesses, it may also reduce the trial and error process for finding effective medication that many patients experience [5]. Oftentimes, these medications have adverse side effects that can impair a patients everyday lifestyle [9]. Computational psychiatry can directly reduce the trial and error cycle by creating a recipe for the target loci [5].
Aside from emotional and biological symptoms, computational psychiatry has the potential to discover and eventually integrate environmental causes of mental illness into diagnosis [5]. Computational psychiatry also shows promise in studying other neurological conditions such as autism spectrum disorder, ADHD, Parkinson’s, and Tourette’s syndrome [3]. Overall, there are many ways machine learning can improve diagnosis and treatment of mental illness and other neurological conditions, allowing psychiatry to evolve past its origins. Analyzing as many factors as possible will lead to more accurate diagnoses and a deeper understanding of each mental illness. Hopefully, it will also reduce or eliminate the trial and error that patients often face when seeking psychiatric help, whether that is determining a patient’s illness or searching for effective medications. Considering the accomplishments of analyzing schizophrenia with computational psychiatry, algorithms for other mental illnesses such as depression, anxiety, or bipolar disorder need to be developed and tested so they can be implemented into diagnosis.
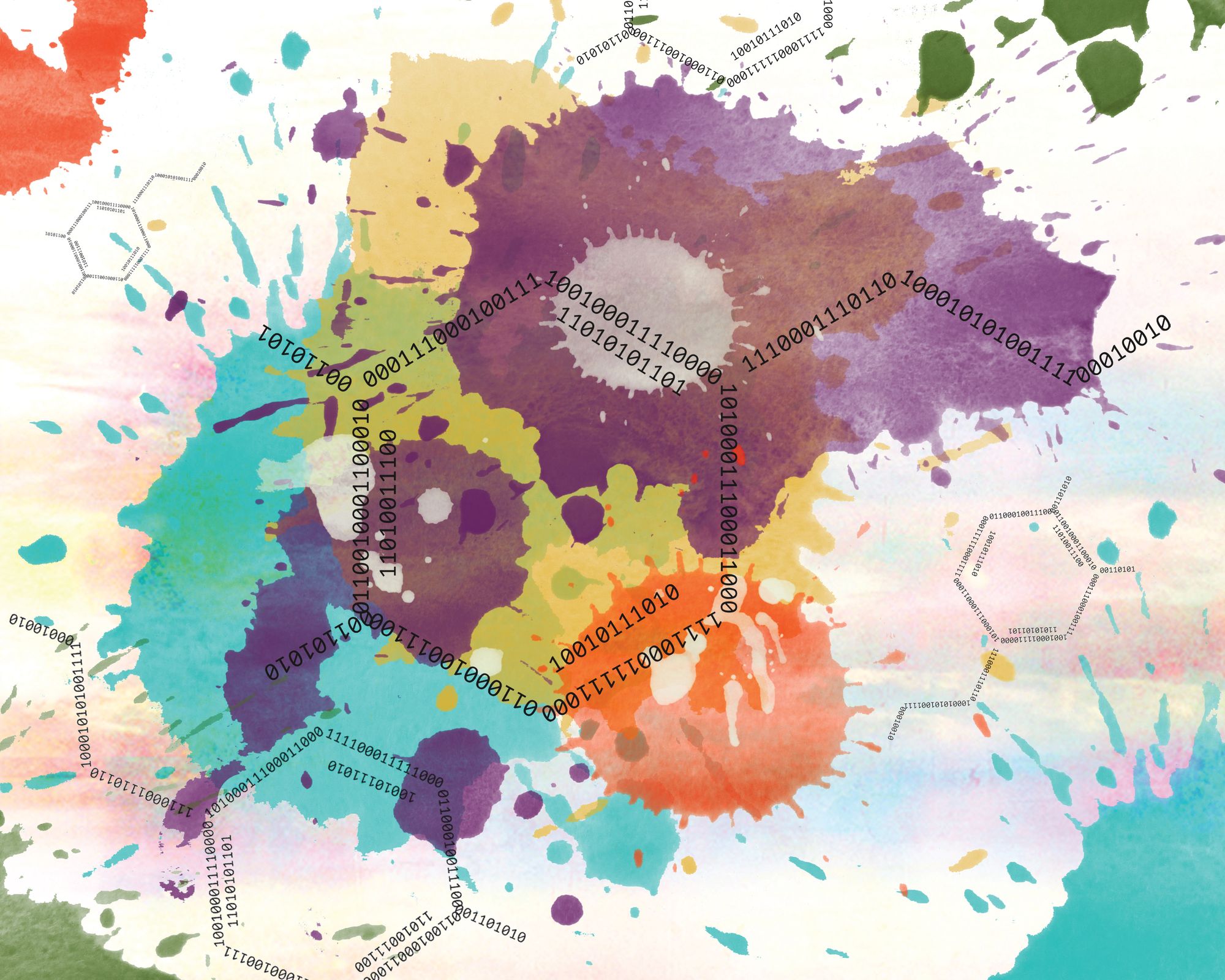
References
- Blashfield, R. K., Keely, J. W., Flanagan, E. H., & Miles, S. R. (2014). The cycle of classification: DSM-I through DSM-5. The Annual Review of Clinical Psychology, 10, 25-51. https://doi.org/10.1146/annurev-clinpsy-032813-153639
- Wang, X., & Krystal, J.H. (2014). Computational psychiatry. Neuron, 84, 638-654. https://doi.org/10.1016/j.neuron.2014.10.018
- Adams, R., Huys, Q. J. M., & Roiser, J. P. (2015). Computational psychiatry: towards a mathematically informed understanding of mental illness. Journal of Neurology, Neurosurgery, and Psychiatry. https://doi.org/10.1136/jnnp-2015-310737
- American Psychiatric Association. (2013). Diagnostic and statistical manual of mental disorders (5th ed.). Washington, DC.
- Iniesta, R., Stahl, D., & McGuffin, P. (2016). Machine learning, statistical learning and the future of biological research in psychiatry. Psychological Medicine, 46(12), 2455-2465. https://doi.org/10.1017/S0033291716001367
- Ikegawa, S. (2012). A short history of the genome-wide association study: Where we were and where we are going. Genomics & Informatics, 10(4), 220-225. https://doi.org/10.5808/GI.2012.10.4.220
- Schizophrenia Working Group of the Psychiatric Genomics Consortium. (2014). Biological insights from 108 schizophrenia-associated genetic loci. Nature, 511, 421–427. https://doi.org/10.1038/nature13595
- Silva, R. F., Castro, E., Gupta, C. N., Cetin, M., Arbabshirani, M., Potluru V. K., . . . Calhoun V. D. (2014). The tenth annual MLSP competition: Schizophrenia classification challenge. 2014 IEEE International Workshop on Machine Learning for Signal Processing (MLSP). https://doi.org/10.1109/MLSP.2014.6958889
- Cascade, E., Kalalii, A. H., & Kennedy, S. H. (2009). Real-world data on SSRI antidepressant side effects. Psychiatry (Edgmont), 6(2), 16-18. https://www.ncbi.nlm.nih.gov/pmc/articles/PMC2719451/