Introduction
Let’s say someone asked you to memorize a random list of twenty animals. Your first thought, aside from “Why?”, would probably be, “How can I manage to memorize all of these creatures?” A strategy called the journey method might be of use to you. Often used by professional mnemonists, the journey method is a memorization technique in which you place the items you need to memorize in various locations along an imaginary familiar route. Mammals aren’t that great at memorizing long strings of numbers or sequences, but tend to be exceptionally talented at memorizing layouts and spatial maps. To harness this ability, imagine a familiar route that you know, such as a layout of rooms in your home, and picture the animals along it. You might place an elephant in your bathroom, a lion in your bedroom, a giraffe in your living room, and a cat in the study. By mentally traversing the route, you can recall the particular animals in their locations.
Our ability to memorize spatial maps so well has been attributed to the close association between the hippocampus, a brain region critical to episodic memory, and the entorhinal cortex, a region that is involved in spatial reasoning. In order to understand how spatial memory is formed, we’ll need to dive deep into the entorhinal cortex to discuss the variety of cell types involved, how they are thought to represent spatial mapping, and how this representation connects to episodic memory.
Discovery
In the late 1960s, neuroscientist John O’Keefe, while searching for an entirely different region of a rat’s brain, accidentally poked his electrode into the cortex just outside the hippocampus. He found that a hippocampal neuron he recorded from fired more the faster the animal ran, which was thought to be unrelated to hippocampal function [1]. This “speed cell” is part of what later came to be called the medial entorhinal cortex (MEC), and it runs directly alongside the length of the hippocampus. O’Keefe soon also discovered “place” cells in the MEC that fired when the rat was in specific locations. Later research found that another type of cell in the MEC, called a grid cell, fires in response to a grid of locations in space, similar to points on a coordinate plane [1]. We now also know of two additional cell types: border cells, which fire in response to any kind of environmental border (both walls and steep drops) and head direction cells, which fire depending on which direction an animal is facing [2]. The MEC is thought to model an animal’s location using these space- and orientation-representing cells (which receive sensory feedback) as the animal moves around a space [2]. While they may not be responsible for memory formation, the representations that these cell types help construct form an important piece of the content spatial memories store.
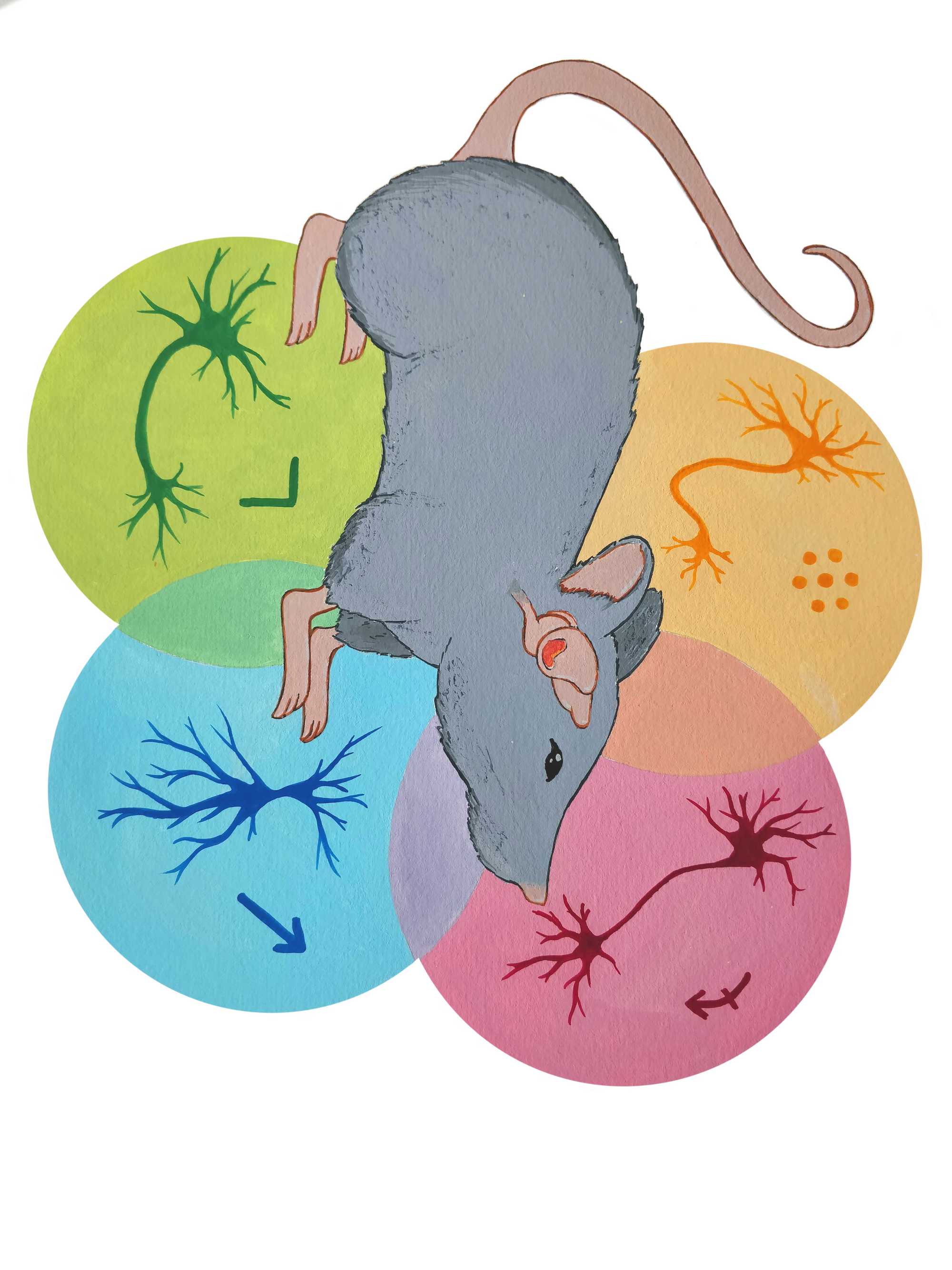
What Is a Cell Type?
As important to spatial representation as they are, the four cell types described above only make up around 41 percent of MEC cells [2]. The other 59 percent were left unclassified, leaving open the question of how the rest of the MEC contributes to an animal’s experience.
To understand why MEC cell classification has been so limited, we’ll need to dive deep into neuron classification and what it really means to be a certain cell type. Scientists can classify neurons in a variety of ways. The MEC neurons previously described were classified functionally, or based on what they fire in response to [2]. In order for these classification methods to be valid, neurons of the same type need to be more similar to one another than they are to neurons of other types [2]. Speed, grid, border, and head direction cells in the MEC are classified by analyzing firing patterns. For example, to identify grid cells, scientists calculate a grid score, which is a measure of how grid-like a neuron’s firing patterns are. Scientists then decide that cells with a grid score higher than some arbitrary number, or threshold, are grid cells and cells that don’t have high enough grid scores are not. Speed, border, and head direction cells are classified in a similar way [2].
However, since the thresholds for these cell-type scores are somewhat arbitrary, they don’t always reflect the biological patterns of neurons [2]. Part of the versatility of the human brain lies in its ability to generalize, that is, to be able to use the same neurons to perform many different tasks. In generalized brain areas that perform a variety of functions, neuroscientists find that single neurons fire in response to a seemingly random set of events during tasks such as sensation, cognition, and motion. This defies the notion that one neuron fires in response to only one stimulus. It is only when recording from populations of neurons that these single neuron firing patterns aggregate to form a firing pattern that makes sense in the context of the task. Since these neurons fire in response to many events, a well-defined functional classification is difficult. Scientists couldn’t be sure what the MEC did because their previous method of classification did not properly reflect the variety of functions entorhinal neurons can have [2]. It is unknown how the MEC would function with only these strictly defined cell types, but it would likely be severely limited in its applications: namely, there would be no way to integrate spatial parameters into a cohesive spatial representation. It is clear that such a system would not lead to the excellent spatial mapping abilities that real animals possess.
Revising Cell Classification
Given that this initial classification method only identified functions of 41 percent of MEC neurons, scientists wondered whether the function of this brain structure might be responsible for more than just encoding spatial maps [3]. In order to understand the functions of more neurons, they chose to attempt a new method of functional classification, in which they collected data about different spatial parameters during a task (head direction, speed, etc.) and assessed which of these parameters best predicted the firing patterns of MEC neurons. If two variables predicted a neuron’s firing better than one, the neuron could be said to fire in response to both of them. Rather than identifying neurons as responding to only one variable, this way of classifying a neuron’s function broadens the definition of neuron function and accounts for neurons that fire in response to many events. Therefore, while still a functional classification, it was revolutionary in that it allowed cells to have classifications other than strict, arbitrarily defined cell types [3].
When researchers applied their method to the MEC, they found that they were able to identify spatial parameters of 71 percent of MEC neurons, corroborating the hypothesis that the vast majority of the MEC deals with spatial modeling [3]. The new method of functional classification included a larger number of MEC neurons, providing us with a richer picture of what cells in the MEC do [3]. Furthermore, with the addition of more generalized cell types, more complex behavior becomes possible. Compared to a hypothetical system with neurons performing singular functions, a more flexible MEC classification seems better suited for spatial mapping.
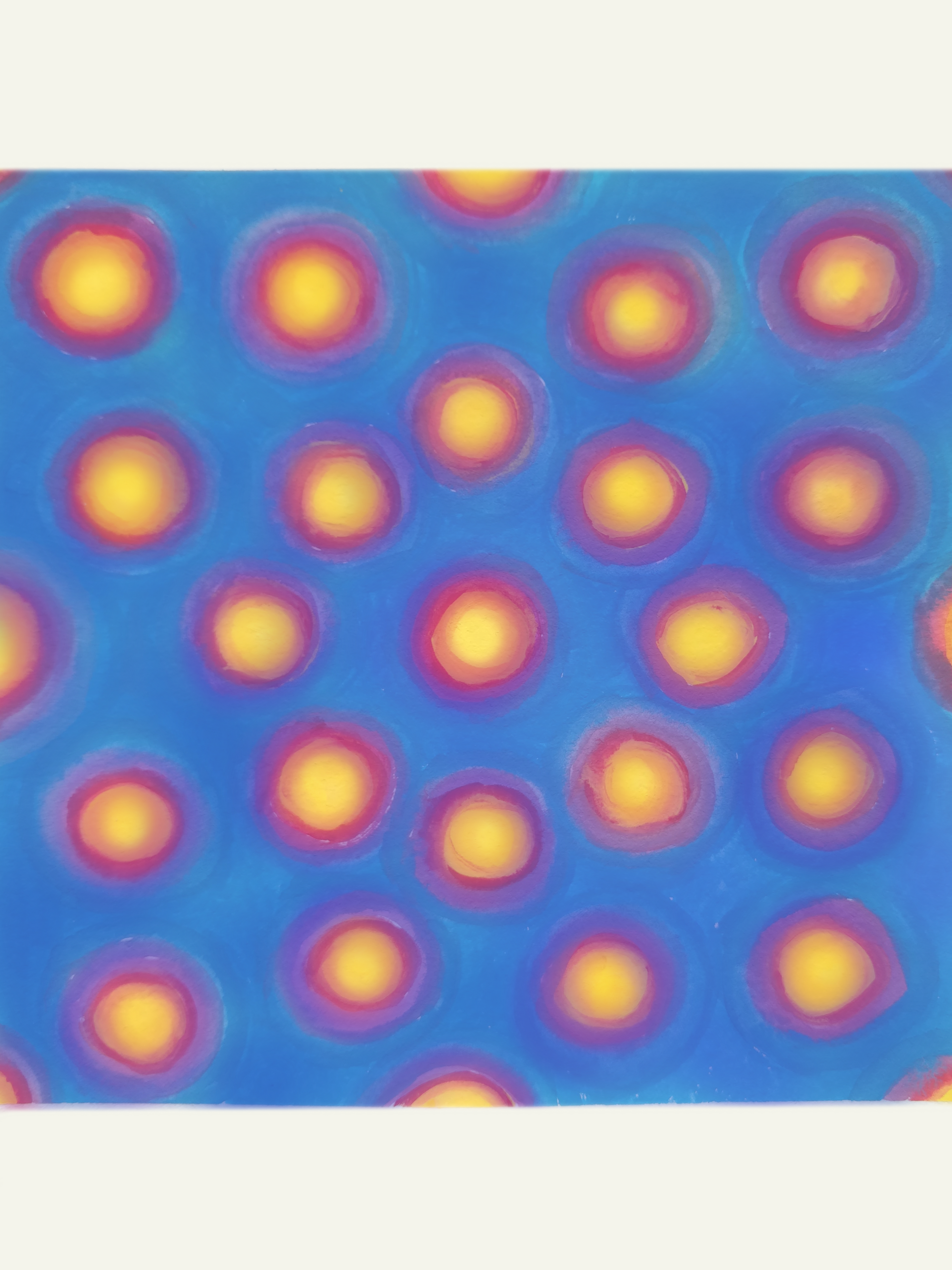
The Function of the MEC
Newer experiments have expanded our understanding of the capabilities of the MEC even further. It has now been shown that neurons in the MEC can change their function based on context, even mapping non-spatial information. In one experiment, scientists compared the firing of various cell types in rats exposed to an environment with and without a rewarded location [4]. They discovered populations of cells that changed their firing patterns to represent the rewarded location when in the rewarded environment [4]. Moreover, some neurons encoded different spatial parameters when the animal ran faster or slower, such as speed and head direction [3]. Thus, the way neurons in the MEC represent an animal’s spatial environment can be dependent on the behavioral context of an animal. In another experiment, rats pressed a lever in response to an increasing pitch and were trained to release it when the pitch reached a certain level. In this case, some MEC neurons fired in response to certain pitches, or certain locations in that pitch space. These experiments suggest that MEC neurons are highly flexible, and can widely vary their firing based on context [2].
Given that the MEC is far more versatile and general than previously thought, some scientists hypothesize that it may encode what they call the “episodic state” of an animal [2]. The episodic state is defined as the minimal amount of information required to predict an animal’s future experience or actions that lead to reward, such as food. If an animal is running in space, spatial parameters will be useful in predicting what it will experience in the future, namely whether it reach a reward location. Alternatively, if it’s doing an auditory task, pitch and lever pressing now become relevant in predicting what future actions will lead to a reward. In essence, the MEC may be crucial to our ability to predict the consequences of our movements and actions, by mapping where we are in a task and where we are going [2]. This insight may help us understand how the journey method maps non-spatial information. The pitch experiment discussed earlier may only deal with sensory information, but perhaps the MEC could be involved in mapping factual information, such as a list of animal names.
A Close Association: The Entorhinal Cortex and the Hippocampus
As we have discussed, the medial part of the entorhinal cortex encodes spatial information about objects; the other half of this region, the lateral entorhinal cortex, may encode certain nonspatial characteristics of objects [5]. Based on experiments in rats, the lateral entorhinal cortex may be required for recognizing objects in new contexts [6]. Both the lateral and medial entorhinal cortex connect to the hippocampus, which some scientists hypothesize then integrates spatial and nonspatial information about objects to give us an understanding of what objects are where [5]. In humans, success at remembering objects in certain places can be somewhat predicted by the sizes of the various parts of the entorhinal cortex and hippocampus [7]. All of this indicates that these two brain regions are crucial to your ability to memorize objects and their locations. The neurons of your entorhinal cortex may allow you to map them, and your hippocampus can then capture this episodic state, and record it as an episodic memory. These neurons may be crucial to your ability to go about your daily business with a memory of where you’ve been and what you did.
Conclusion
The question of how to classify neurons remains a pertinent one for any brain region that neuroscientists might study, as the function of a brain region is the function of its neurons. When less arbitrary and more inclusive classification methods are implemented, we can more effectively learn what regions in the brain do. From the initial discovery of strictly defined spatial cell types to the discovery of the MEC’s far more versatile capabilities, this small but essential brain structure is receiving intense attention in current research. Scientists continue to explore how episodic states might be encoded by the entorhinal cortex and what other, higher-order functions it may have in conjunction with the hippocampus.
References
[1] O'Keefe, J. (2014). Spatial cells in the hippocampal formation. Nobel Lecture on 7 December 2014 at Aula Medica, Karolinska Institutet in Stockholm, http://www.nobelprize.org/mediaplayer/index.php?id=2413.
[2] Hardcastle, K., Ganguli, S., & Giocomo, L. M. (2017). Cell types for our sense of location: where we are and where we are going. Nature Neuroscience, 20(11), 1474–1482. doi:10.1038/nn.4654
[3] Hardcastle, K., Maheswaranathan, N., Ganguli, S., & Giocomo, L. M. (2017). A multiplexed, heterogeneous, and adaptive code for navigation in medial entorhinal cortex. Neuron, 94(2). doi:10.1016/j.neuron.2017.03.025
[4] Butler, W. N., Hardcastle, K., & Giocomo, L. M. (2019). Remembered reward locations restructure entorhinal spatial maps. Science, 363(6434), 1447–1452. doi:10.1126/science.aav5297
[5] Hargreaves, E. L., Rao, G., Lee, I., & Knierim, J. J. (2005). Major dissociation between medial and lateral entorhinal input to dorsal hippocampus. Science, 308(5729), 1792–1794. doi:10.1126/science.1110449
[6] Wilson, D. I., Langston, R. F., Schlesiger, M. I., Wagner, M., Watanabe, S., & Ainge, J. A. (2013). Lateral entorhinal cortex is critical for novel object-context recognition. Hippocampus, 23(5), 352–366. doi:10.1002/hipo.22095
[7] Yeung, L.-K., Olsen, R. K., Hong, B., Mihajlovic, V., Dangelo, M. C., Kacollja, A., … Barense, M. D. (2019). Object-in-place memory predicted by anterolateral entorhinal cortex and parahippocampal cortex volume in older adults. Journal of Cognitive Neuroscience, 31(5), 711–729. doi:10.1162/jocn_a_01385