Introduction
Picture yourself sitting in a coffee shop. When the barista sets a drink down on the counter, you probably don’t expect the drink to fall through the counter’s surface or bounce off the counter into the air. If the barista slides one cup along the counter and it bumps into another cup that doesn’t budge, you might guess that the cup that does not move has a larger mass. Similarly, if someone attempted to stack many coffee cups on top of one another, you will likely be able to quickly predict whether or not it will topple as they walk away. As humans, we make countless split-second predictions about our physical surroundings each day, often without even realizing it. Unravelling the mechanisms of this physical reasoning, however, has puzzled scientists for decades. Only recently, alongside advancements in the field of artificial intelligence, have scientists begun to understand the massive scope of neural circuitry that may underlie these seemingly simple physical judgements [1].
Early Studies on Physical Reasoning
To understand how we perceive the world around us, we must first consider the difference between the knowledge humans have at birth and the knowledge acquired through living. Recent research on the infant brain increasingly supports a theory known as the core knowledge thesis [1]. According to this theory, infants are born with a certain set of core principles that are then built upon over time as they acquire new knowledge. The remarkable speed and regularity with which infants piece together the physical laws governing the world around them has led scientists to believe a set of core physical intuitions exists innately within the human brain [2]. For example, most infants understand that objects exist even when they aren’t visible, a concept known as object permanence. Furthermore, five-month-old infants can consistently predict the different behaviors of solid and non-solid substances [2,3]. Early comprehension of these core physical properties points to the importance of being able to accurately perceive our physical surroundings, even early on in child development. Because infants uniformly and rapidly develop an understanding of physical phenomena, it is believed that these physical intuitions, upon which all later physical reasoning is built, are either innate at birth or developed very early on in life.
The core knowledge theory is a relatively recent scientific development. Early behavioral research initially suggested that babies, and even adult humans, were surprisingly bad at predicting physical outcomes [1]. Years after these initial experiments, it was revealed that this poor performance was the result of testing conditions and not a lack of physical reasoning. These early experiments usually involved giving participants a hypothetical scenario and asking them to describe what would happen next. A common exercise asked participants to draw on a sheet of paper the path of an object that had just exited a curved tube. An overwhelming majority of participants drew a curved line when in reality the object continues along a straight trajectory. Another task asked participants to draw the path of an object dropped from a moving source, like an airplane. In this case, the majority of participants drew the path of the object straight downward when it would actually demonstrate projectile motion. Because of the discrepancies between the actual behaviors of these objects and their perceived behaviors by the participants, early scientists concluded that humans possessed little, if any, physical intuition. Only within the past decade have these misconceptions been explained, and a new frontier of understanding has emerged. It is now known that humans are not great at conjuring up physical predictions on paper, but are actually remarkably accurate when asked to make similar predictions in more familiar contexts.
When the same knowledge was tested in more familiar contexts, the vast majority of participants were able to accurately predict physical outcomes. For example, one study asked participants both to draw the path of a ball exiting a curved tube and choose where to stand in order to catch a ball exiting the same tube [4]. Only 7% of participants in the study could accurately draw the ball’s path, but almost 50% were able to correctly predict the path by choosing where to stand in order to catch it. In the case of an object falling from a moving source, researchers realized that if the question was posed in the participants’ frame of reference, the majority of people were suddenly able to predict the correct outcome [1]. Instead of asking where a box would land if a flying airplane dropped it, for example, researchers asked where a ball would land if the participant were to drop it while walking. In this context, there were few discrepancies between the actual physical behaviors of the objects and their predicted behaviors. This demonstrates the importance of physical perceptions as they relate to everyday experience, rather than the high-level concepts and on-paper equations many people might imagine when they think of physics as a subject.
Early on, another intriguing line of evidence involving gravity was discovered that further supported the existence of certain innate physical reasoning centers in the brain. As humans, we are all familiar with gravity and its effects on us and the objects we interact with daily. But fifteen years ago, research on astronauts provided the first evidence that Earth’s gravity may be more hardwired in the human brain than originally thought [5]. Researchers found that part of the vestibular cortex – a region responsive to input from the inner ear, and known to be involved with balance and spatial orientation – was active only under the effects of Earth’s specific gravity, and not in the zero-gravity conditions of outer space. In a related line of research, scientists investigated the potential effects of gravity perception on spatial orientation and physical reasoning. Scientists discovered that humans could quickly solve problems requiring them to predict the angle at which a glass of water would spill while they were sitting or standing, but they had significant trouble solving the same problem while laying down. These results may be explained by certain patterns of brain activity, associated with gravity perception and spatial orientation, being present only in frequently-experienced scenarios and not more unnatural ones [6]. The fact that participants performed poorly only when their spatial orientation was altered suggests that certain physical reasoning tools, like those associated with gravity in the vestibular cortex, are only available when the scenarios for their use are sufficiently familiar and practical. Because, in the experimental setting, the participants are trying to pour the glass from an abnormal position, the vestibular cortex may not be engaged enough to complete the task with precision and accuracy [6]. These studies together provide strong evidence for a highly evolved human processing system for at least one physical property, gravity, and it is possible that others exist and will continue to be discovered by future research.
The experiments described so far demonstrate how some physical reasoning may be innate by asking subjects to predict outcomes in the real world. Recent research from Harvard and UPenn though has suggested that this type of innate physical understanding even extends into the realm of imagination [7]. The researchers conducting this study described imaginary magical spells and asked their participants to rank the estimated difficulty of performing them. While this may seem silly on the surface, what they found was that nearly all participants reliably ranked spells that violate our native physical laws as harder on the difficulty scale. For example, the spell reliably rated as the easiest to perform was changing the color of a frog from green to purple. The researchers believe that since changing color is not an unfamiliar physical occurrence, and because color is not necessarily an essential property of the object, these spells are easier to imagine. The spell consistently ranked as the hardest was conjuring up a frog from thin air. The researchers believe that since creating mass from nothing is something humans never physically observe, it is much harder to imagine performing this spell. This research highlights how people use their intuitive understanding of the physical world to reason about all things, real or imaginary [7]. Because physical reasoning was used to understand these completely novel magical spells, this study also provides additional evidence to suggest that our physical reasoning is, at least on some level, innately present, even when used subconsciously or with tasks that have never been explicitly observed.
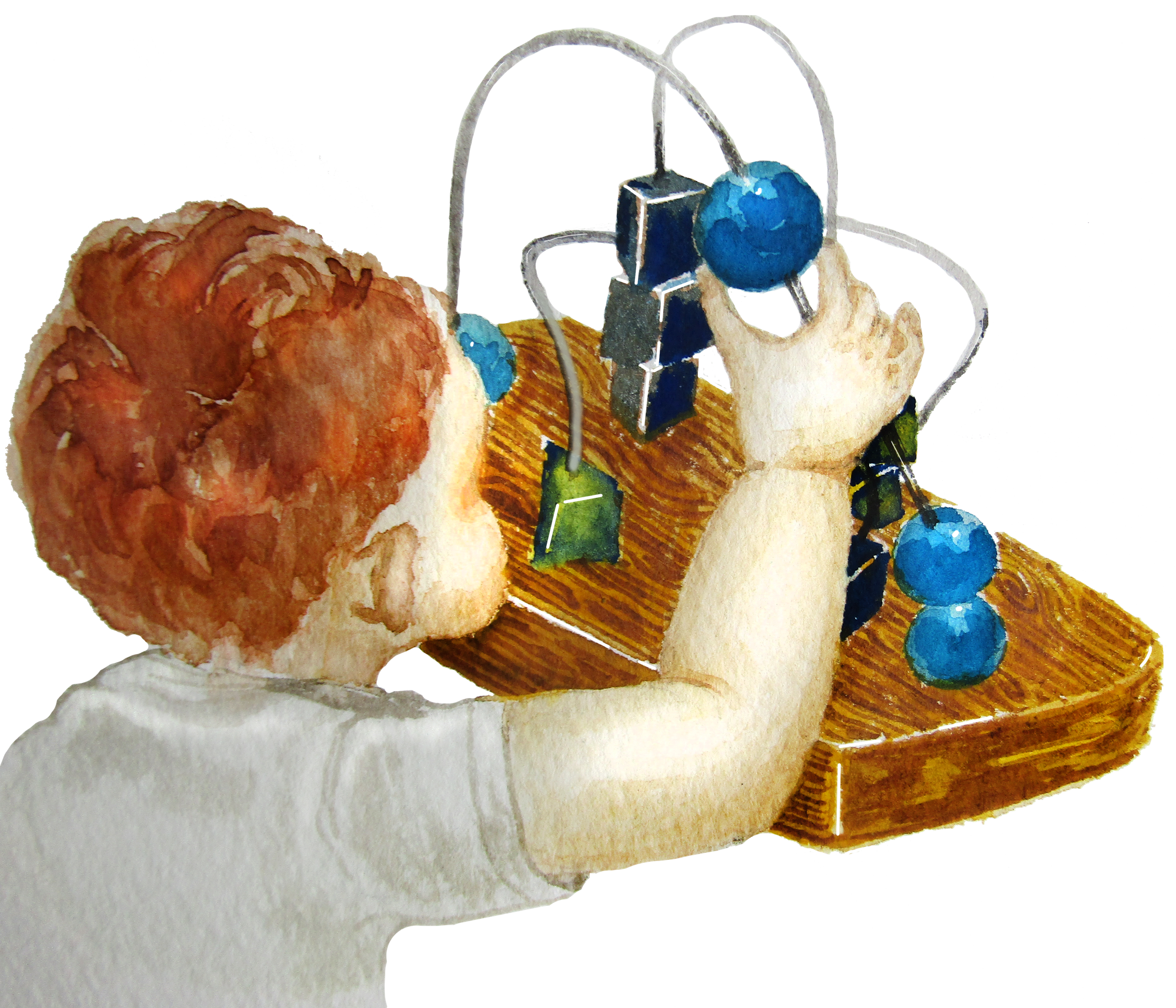
The Current Model
As evidence increasingly points toward the existence of an innate physical reasoning system in humans, the location and mechanism of this system in the brain remains, for the most part, a mystery. The earliest theory was that humans have a neural structure that measures certain key physical variables, like the initial velocity of an object seen falling. This structure, or a related one, was then thought to perform subconscious, rapid, and flawless calculations of traditional physics equations to arrive at a final prediction [8]. These theories were mostly disproven when it was discovered that human measurements of physical variables, like exact initial and final velocities, from visual information are too inaccurate and unreliable to forecast the predictions people are able to successfully make each day. Humans are not capable of estimating physical data from sensory information precisely enough for this theory to be plausible. Experiments where participants were asked to catch balls while blindfolded also demonstrated that the sensory input that would underlie these estimates is not required for physical reasoning tasks [5]. Before the blindfolding, participants were only shown where the ball was to be dropped from. They were then blindfolded and given an auditory signal when the ball was about to be dropped. The vast majority of participants were able to successfully catch the ball despite the lack of any visual input. This cast even more doubt on the theory that humans are always subconsciously calculating the outcome of physical situations using their visual input to measure relevant variables like velocity and acceleration.
A competing theory posited that humans make physical predictions based on heuristics, or mental shortcuts. Instead of doing any subconscious calculations, a person simply learns patterns from observations early in life, like that when two objects collide, the lighter one moves away faster. They then apply this rule when trying to reason through any situation involving two colliding objects. While this theory explains peoples’ predictions in specific common scenarios, it fails to explain why people are able to rapidly reason through completely novel physical situations and still accurately predict outcomes. For instance, it cannot explain how people are able to accurately predict how much to tilt a glass full of liquid in order to get it to spill, a situation they are unlikely to encounter often enough to form specific rules about. There are also situations in which two contradictory shortcuts might exist, and this theory does not explain how one might be chosen over the other. For example, if someone learns that a certain heavy object, like a large boulder, produces an unstoppable force when moving, they could predict that this object will always move an object it collides with simply by virtue of being unstoppable. But suppose they also learned of a different object, like a giant wall, with a mass so great that the object is essentially immovable. With only these two shortcuts, it becomes impossible to predict what will happen when the object with unstoppable force meets the immovable object. For many years, researchers went back and forth on these heuristic-based theories, unable to find evidence that could explain how heuristics alone could account for the range of novel physical problems humans are capable of analyzing [8].
Alongside rapid advancements in the fields of artificial intelligence and video game development, researchers have developed a new, more complete theory of how physical reasoning may work in the human brain [1,9]. By trying to design artificial intelligence systems that recreate the experience of living in and perceiving the physical world, researchers were able to make hypotheses about what is occurring in the human brain during similar processes. Over the past decade, one computational model has emerged that accurately recreates human predictions about the outcome of a wide variety of physical scenarios using complex mathematical tools [1]. This model is based on the brain’s ability to come up with mental simulations and use them to make predictions [6]. There is strong evidence suggesting that humans heavily incorporate some kind of mental simulation into their problem-solving, especially with regards to physics problems. In one study, participants were shown a set of three pulleys and asked how the middle one behaved. Every participant first looked at the top pulley, then the middle, then the bottom. This order is critical because it demonstrates a step-wise approach to solving the problem that is highly indicative of creating a mental simulation of the scene. Some participants further described their mental simulation of the system explicitly, and others used gestures to illustrate a similar simulation process. In some ways, this simulation theory seems obvious – anyone who has had to reason through a physical problem can probably remember playing some kind of mental video of the scene in their head. But this experience alone does not explain how or why we rely on these simulations to make physical predictions [6].
To successfully capture human brain performance during physical reasoning tasks, this model must include not just any simulation, but one that simultaneously incorporates other relevant factors, like uncertainty [10]. For example, imagine having to determine which of two boxes was heavier based on vision alone. This is impossible because mass is a physical characteristic that is unseen. But it has previously been shown that humans are able to incorporate both seen properties, like height, and unseen properties, like mass, into their physical predictions. Computational models were able to replicate this by incorporating a determined amount of uncertainty into their calculations: the more unseen variables there are, the more uncertain the outcome.
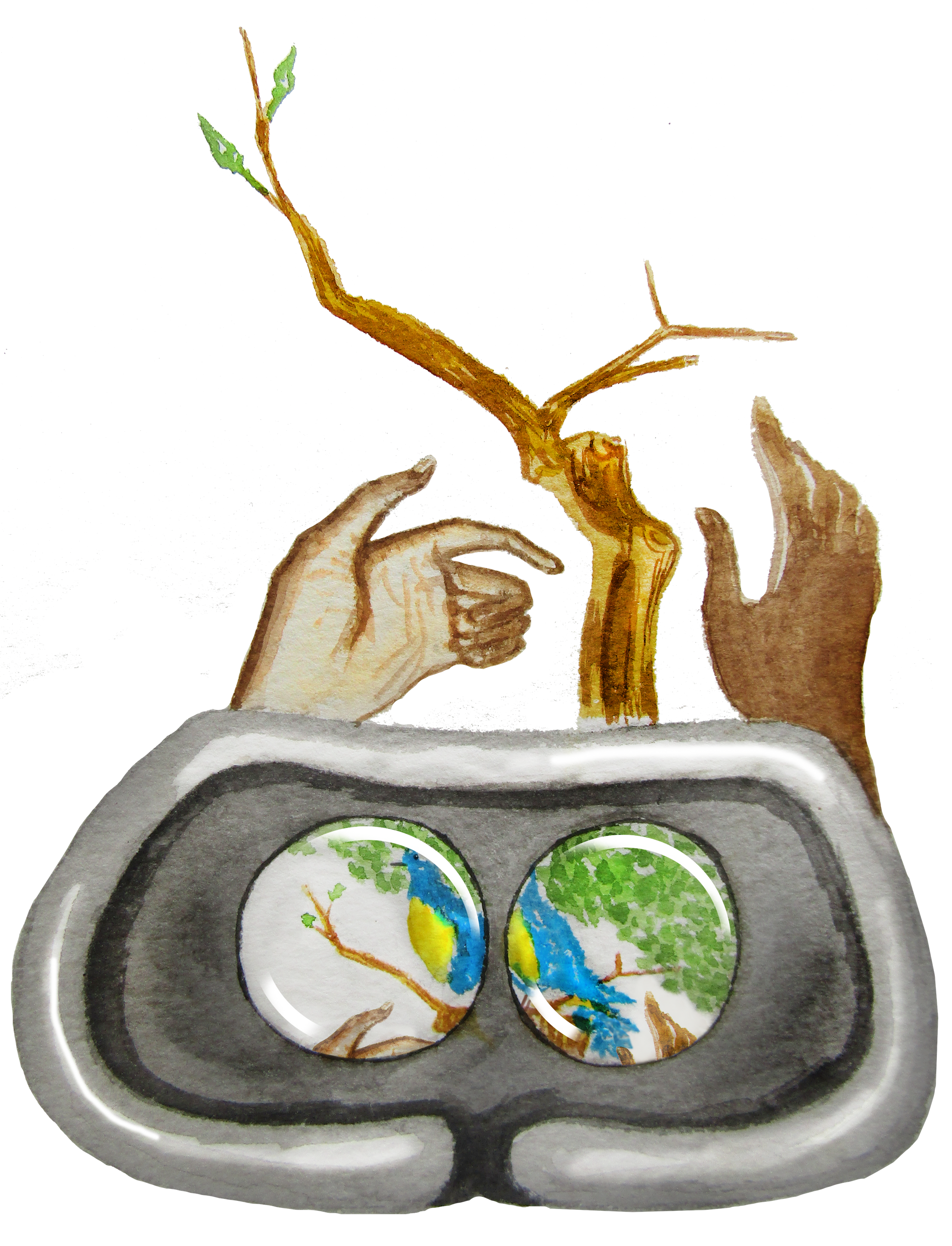
Like the human brain, these models do not require the explicit calculation of any physical variables or equations, but are instead based purely on estimates of probability [10]. If given a situation where there are ten possible outcomes, the model would predict, based on background information and knowledge of probabilities, which of the ten outcomes is most likely to occur. This background information in humans is thought to be the innate physical knowledge we are born with or acquire within the first year of life. The knowledge of certain probabilities is thought to come from our experiences with the physical world. If we realize, for example, that stacking dishes a certain way causes them to fall every single time, we know there is a high probability of that outcome in that given scenario. Without any input, the model predicts that any outcome is equally probable. As more data is gathered, and more outcomes are observed, the probability is adjusted. This model, known as the “Noisy Newton” model, has been successfully applied to a huge variety of physical scenarios with a remarkable likeness to the accuracy of human reasoning in the same physical scenarios [11, 12, 10]. Researchers across the world have used it to accurately reflect human predictions of physical outcomes, but also the changes in these predictions over time as more information is gathered and knowledge expands [10]. This makes this model an exceptional tool for understanding how our brains may be parsing and reasoning with the physical world we live in.
With multiple lines of evidence pointing towards the existence of some kind of innate physics engine in humans, some researchers have wondered if it is possible to pinpoint its location in the brain [9]. By monitoring neural activity while people completed various physical-reasoning tasks, researchers found hotspots that were significantly more active during physical scene analysis, like examining the stability of a tower of blocks, than they were during other related activities, like describing the colors of the blocks present in the same scene. The researchers observed increased activity specific to physical reasoning problems in regions of the brain involved with motor planning behaviors and sensory processing, like the premotor cortex, the parietal lobe, and the left supramarginal gyrus. These hotspots are believed to form a network in the brain that helps humans reason through their physical environments. Because these regions are not all located in the same area, it has recently been proposed that a multiple demand network is involved in many physical reasoning processes. A multiple demand network is a set of many brain structures thought to be involved with processing a wide range of inputs and behaviors at one time. Currently, the regions implicated in these physical reasoning tasks are thought to work together, each functionally behaving most similarly to the regions of the brain involved in motor planning and object visualization [9]. This makes sense when considering the highly mechanical and visual nature of the physical problems and inputs in the world around us. As we continue to unveil exactly how our brains perceive the physical world, we will also continue to progress our understanding of the brain structures involved in this perception.
While the development of certain computational models has significantly progressed our understanding of physical reasoning, more research is needed to explain exactly how these models correlate to physical structures in the human brain. In order to understand the mechanisms that underlie even basic interactions with our physical surroundings, it will also be necessary to explore how these complex and thorough simulations are initially formed in the infant brain. In the future, understanding innate physical reasoning could be used to dramatically improve physics education programs by teaching the subject in more intuitive ways. Another fun consequence of these physical prediction studies is the improved knowledge available to video game developers that can help them design their game characters to apply the laws of physics like real humans, creating a more realistic game as a result. On a more serious note, knowing where we organize physical reasoning in the brain may eventually lead to treatments for disorders related to physical perceptions, like vertigo or the side-specific spatial neglect that often occurs after a stroke. A more complete understanding of human physical reasoning will not only teach us about our perceptions of the physical reality we find ourselves in, but also about the very way we learn about and reason through what is happening in the world around us, from developing flying airplanes and rockets to knowing which towers of coffee cups are likely to topple.
References
- Kubricht, J. R., Holyoak, K. J., & Lu, H. (2017). Intuitive Physics: Current Research and Controversies. Trends in Cognitive Sciences, 21(10), 749–759. doi: 10.1016/j.tics.2017.06.002
- Baillargeon, R., & Carey, S. (2012). Core cognition and beyond: the acquisition of physical and numerical knowledge. Cambridge: Cambridge University Press.
- Hespos, S. J., Ferry, A. L., Anderson, E. M., Hollenbeck, E. N., & Rips, L. J. (2016). Five-Month-Old Infants Have General Knowledge of How Nonsolid Substances Behave and Interact. Psychological Science, 27(2), 244–256. doi: 10.1177/0956797615617897
- Smith, K. A. et al. (2013). Consistent physics underlying ballistic motion prediction. Proceedings of the 35th Annual Conference of The Cognitive Science Society, pp. 3426-3431.
- Zago, M., & Lacquaniti, F. (2005). Visual perception and interception of falling objects: a review of evidence for an internal model of gravity. Journal of Neural Engineering, 2(3). doi: 10.1088/1741-2560/2/3/s04
- Hegarty, M. (2004). Mechanical reasoning by mental simulation. Trends in Cognitive Sciences, 8(6), 280–285. doi: 10.1016/j.tics.2004.04.001
- Mccoy, J., & Ullman, T. (2019). Judgments of effort for magical violations of intuitive physics. Plos One, 14(5). doi: 10.1371/journal.pone.0217513
- Sanborn, A. N., Mansinghka, V. K., & Griffiths, T. L. (2013). Reconciling intuitive physics and Newtonian mechanics for colliding objects. Psychological Review, 120(2), 411–437. doi: 10.1037/a0031912
- Fischer, J., Mikhael, J. G., Tenenbaum, J. B., & Kanwisher, N. (2016). Functional neuroanatomy of intuitive physical inference. Proceedings of the National Academy of Sciences, 113(34). doi: 10.1073/pnas.1610344113
- Hamrick, J. B., Battaglia, P. W., Griffiths, T. L., & Tenenbaum, J. B. (2016). Inferring mass in complex scenes by mental simulation. Cognition, 157, 61–76. doi: 10.1016/j.cognition.2016.08.012
- Bates, C. J., Yildirim, I., Tenenbaum, J. B., & Battaglia, P. (2019). Modeling human intuitions about liquid flow with particle-based simulation. PLOS Computational Biology, 15(7). doi: 10.1371/journal.pcbi.1007210
- Battaglia, P., Hamrick, J., & Tenenbaum, J. B. (2013). Simulation as an Engine of Physical Scene Understanding. PsycEXTRA Dataset. doi: 10.1037/e633262013-904