Over the last fifteen years, brain-computer interfaces (BCIs) – computerized systems that allow the brain to control external devices – have gone from the imaginative realm of science fiction to real world science fact.
How do BCIs work?
BCIs are based off the idea that there is a physical basis in the brain for every thought, action, and behavior that we can perform. This idea serves as the foundation for most modern cognitive neuroscience research, and is generally accepted by the scientific community. There is some disagreement as to whether the physical brain is the sole basis of consciousness or if there is another separate non-physical ‘mind’ driving the brain (Dualism vs. Monism). However there is a large amount of compelling evidence that for certain specific tasks, movements, thoughts, or processes there are analogous specific parts of the brain that are active.
The goal of a BCI is to detect thought-related activity in the brain, and turn it into a signal that can be used by a computer to initiate some task. There are some BCIs that function in the opposite direction, taking a computer generated signal and turning it into something readable by the brain – however for the purposes of this article, the focus will be only on the interactions from the brain to the computer.
At the most basic level, three things are needed to set up a functional BCI: 1) A way of reading input from the brain, 2) A way to extract something recognizable and associated with a meaningful command from that input, and 3) An output device or computer to execute the command (See Fig. 1).
However, while all BCIs share these common traits, there is a great deal of variation in the specific implementation of each of these three things, and each method has its advantages and disadvantages.
Methods of BCI Input
There are a lot of different methods used to acquire input from the brain for use in a BCI, each with tradeoffs in resolution, invasiveness, portability, and information content that are important to consider.
For example, the first BCIs were developed in rats using implanted electrodes in their motor cortex to control a robotic arm. While implanted electrodes have great local resolution, they are limited in their ability to assess more distant areas, and require risky surgical placement.
Non-invasive techniques include BCIs that use functional magnetic resonance imaging (fMRI), a type of neuroimaging technique that measures the oxygen content in the blood in different areas of the brain using powerful magnets.
fMRI is advantageous in that it is relatively low risk (unless you have an implanted metal device that the magnets will interfere with) and has very accurate spatial resolution so you can more accurately pinpoint specific areas in the brain, however it is limited in that it is slower than most other techniques, and requires large, expensive equipment to operate.
Currently, electroencephalography, or EEG, is the most popular method of acquiring BCI input, because it is both non-invasive and portable. EEG measures the electrical activity of the brain using electrodes placed on the scalp.
Unfortunately, because the EEG electrodes get their signal from the electrical activity that comes through the scalp, skull, and protective membranes surrounding the brain, the signal acquired tends to be weak, fairly low resolution, and is easily disrupted by other artifacts.
Despite these drawbacks, researchers have successfully been able to classify several types of event related potentials (ERPs), changes in EEG patterns that occur in response to some kind of stimulus, useful in BCIs.
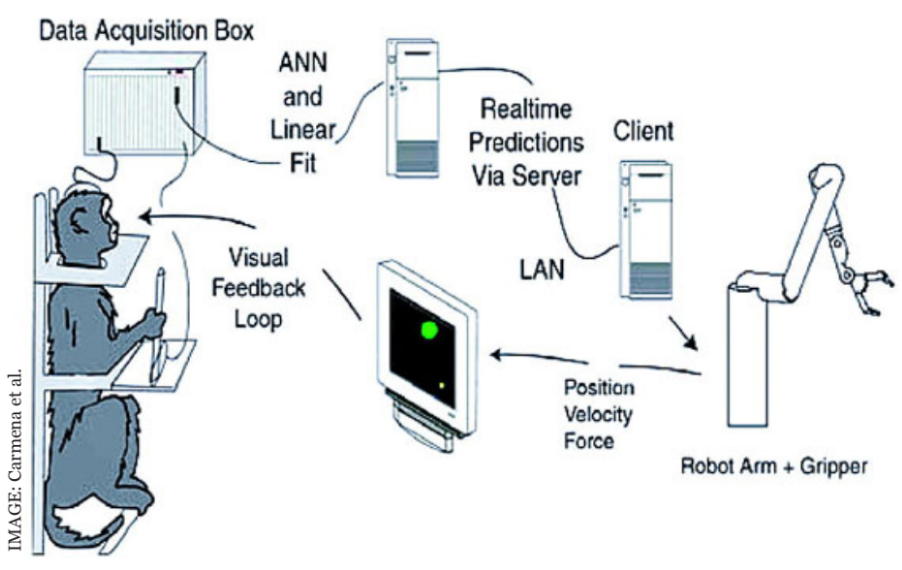
Signal Extraction and Classification
Once real-time data has been acquired from the brain it must be interpreted and turned into meaningful information. This generally involves a fair amount of filtering and data processing in order to extract the features of interest. These features are then analyzed by comparison to a known typical feature associated with a certain activity or signal, and if they’re similar enough, there is a positive recognition of the activity or signal in question, which can then be used to drive the BCI.
This was made possible by a large number of imaging studies that have identified characteristic patterns of activation associated with certain stimuli or actions.
For example, visual evoked potentials (VEPs) are one type of detectable signal that occurs when a subject looks at or attends to a stimulus. Another type of signal is the P300 evoked potential, which is an ‘oddball’ response that occurs approximately 300 ms after a weird or unexpected stimulus is thrown into a series of repeated stimuli.
Most BCIs incorporate user feedback from a training period in their classification process to better identify a user’s specific response, though there are some BCIs that rely on the user learning how to control their brain to produce the maximum response. In either case, the end result is a BCI that can somewhat accurately identify signals from the brain, which it can then use to execute a command.
Putting Your Thoughts to Work
After the difficult task of reading and classifying brain signals, designing an output is a fairly simple task. A computer is programmed to identify a signal X and perform an action Y. This is the case for a P300 spelling BCI.
A matrix of letters is presented on a screen, and the rows and columns of letters are flashed in a systematic way so that each letter has a unique set of times when it is flashed. The subject is instructed to concentrate on the letter, and thus when the letter is flashed, a P300 will occur because the flash is an ‘oddball’ stimulus. Therefore there will be a specific expected pattern of P300s for each letter.
If the letter ‘R’ is associated with a P300 response at times a, b, and c, then the BCI can be instructed to output ‘R’ when it registers a P300 at a, b and c.
In comparison to many of the other BCI programs currently available and under investigation, this is a fairly simple example. Computer programmers continue to develop new and innovative ways to use the signals extracted from BCIs to power different devices, such as the partially-autonomous robot controlled with an EEG based BCI created here at the University of Washington.
Present Day BCI Successes
Though there is still a lot of room to improve BCIs, there are a few BCIs that are already commercially available. EEG based games and toys are already on the market by several companies. One Japanese company even produces a set of EEG based kitty ears that perk up in response to an increase in mental activity.
There are also commercially available P300 based BCIs with functions for spelling, painting, and computer control. In medical settings, there are many different clinical trials testing the use of BCIs in a clinical therapies. Currently, however, BCIs have not entered the medical world as an approved intervention.
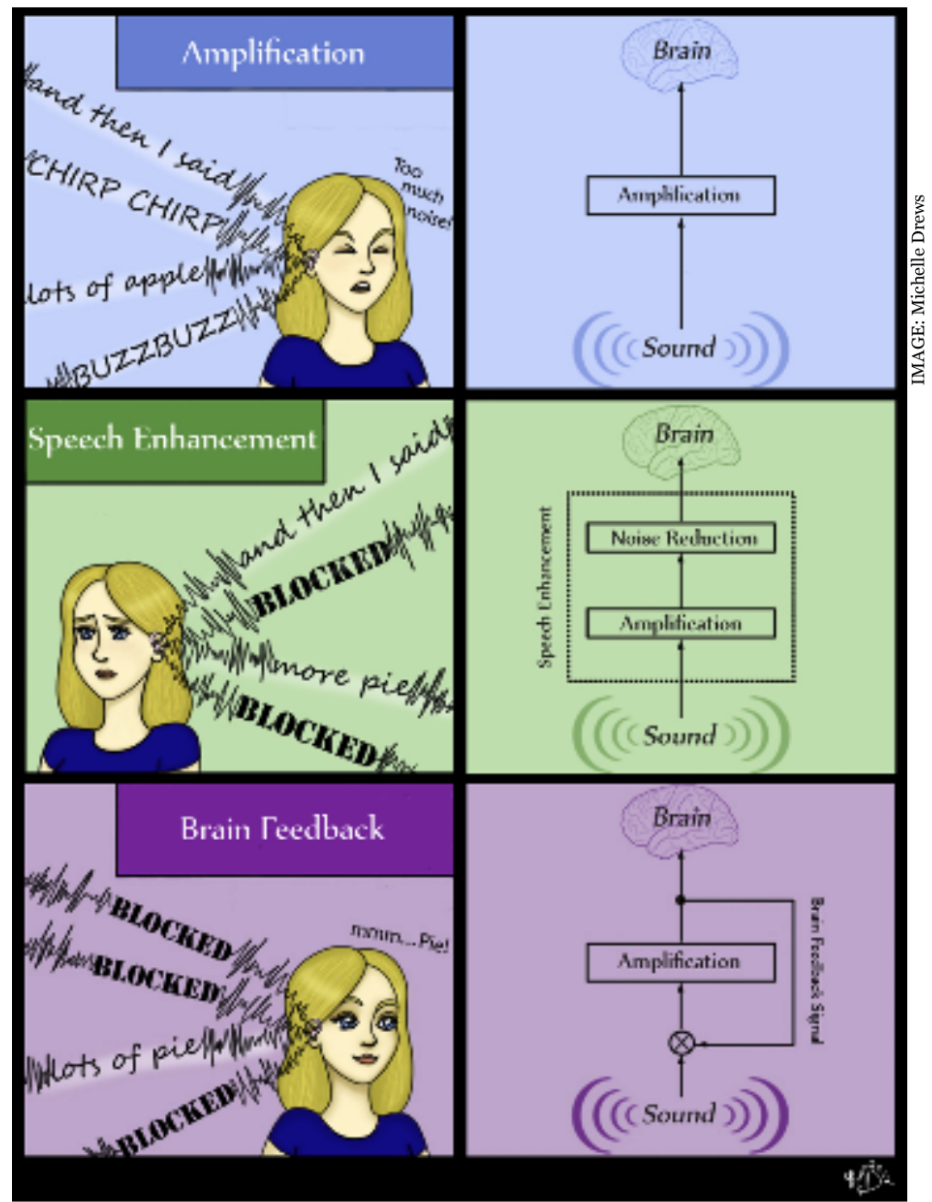
The Future of BCIs
Driven by their widespread potential, BCIs have come a long way in the last two decades. The ideal BCI could help restore functional capability to patients with various disabilities.
Additionally, BCIs could optimize and improve existing technologies such as the hearing aid. Currently, most hearing aids amplify all sound input: a relevant conversation, the hum of the fan, the distant conversation on the other side of the room.
There are some fancier hearing aids that can apply certain filters to their inputs, and filter out sounds that are definitely noise, but that still leaves a problem when there are multiple conversations in the room between which the filter cannot distinguish.
A BCI driven hearing aid may be capable of detecting intended auditory stimulus, such as a conversation someone was attempting to listen to, and selectively amplify that specific conversation.
However, before such technologies become a reality, there is more research that must be done.
Improving the number of reliable meaningful detectable stimuli available for use will be a great advantage in developing a BCI. A BCI able to recognize a certain action or thought pattern won’t be possible without reliable characterizations of exactly what the brain looks like when it is doing that action or thought pattern.
Along these lines, improving methods of classifying brain features to incorporate real-time feedback is also a promising way of increasing BCI functionality.
Finally, working to address the disadvantages associated with the different acquisition methods is also a field of study that could greatly advance BCIs.
It is likely that these devices, once only appropriate in imaginative science fiction, could become a standard integrated part of medicine, communication, and entertainment.