Intro
We all make about 35,000 decisions a day [1]. Whether it’s choosing which coffee shop to visit, deciding between job offers, or considering whether or not to accelerate to catch a yellow light, you are constantly making decisions. But how do these decisions go hand in hand with visual processing?
As you navigate choices every day, what you encounter is processed by the “where pathway” and the “what pathway.” These two information processing streams are overall responsible for vision, attention, and memory, which are important for the process of making a decision. Though these streams are separate, research shows that they may interact with each other [2]. Glass patterns (GPs) are texture stimuli made by randomly placed dots with their accompanied partner dot that can be controlled by a certain protocol rule [3]. However, there are cases where these dots will not have a partner. By using GPs in research, we can study interactions between the two streams. Additionally, they allow researchers to investigate ideas ranging from decision-making to depth perception [3]. This helps us learn more about certain neurodegenerative diseases and contribute to technological advancements.
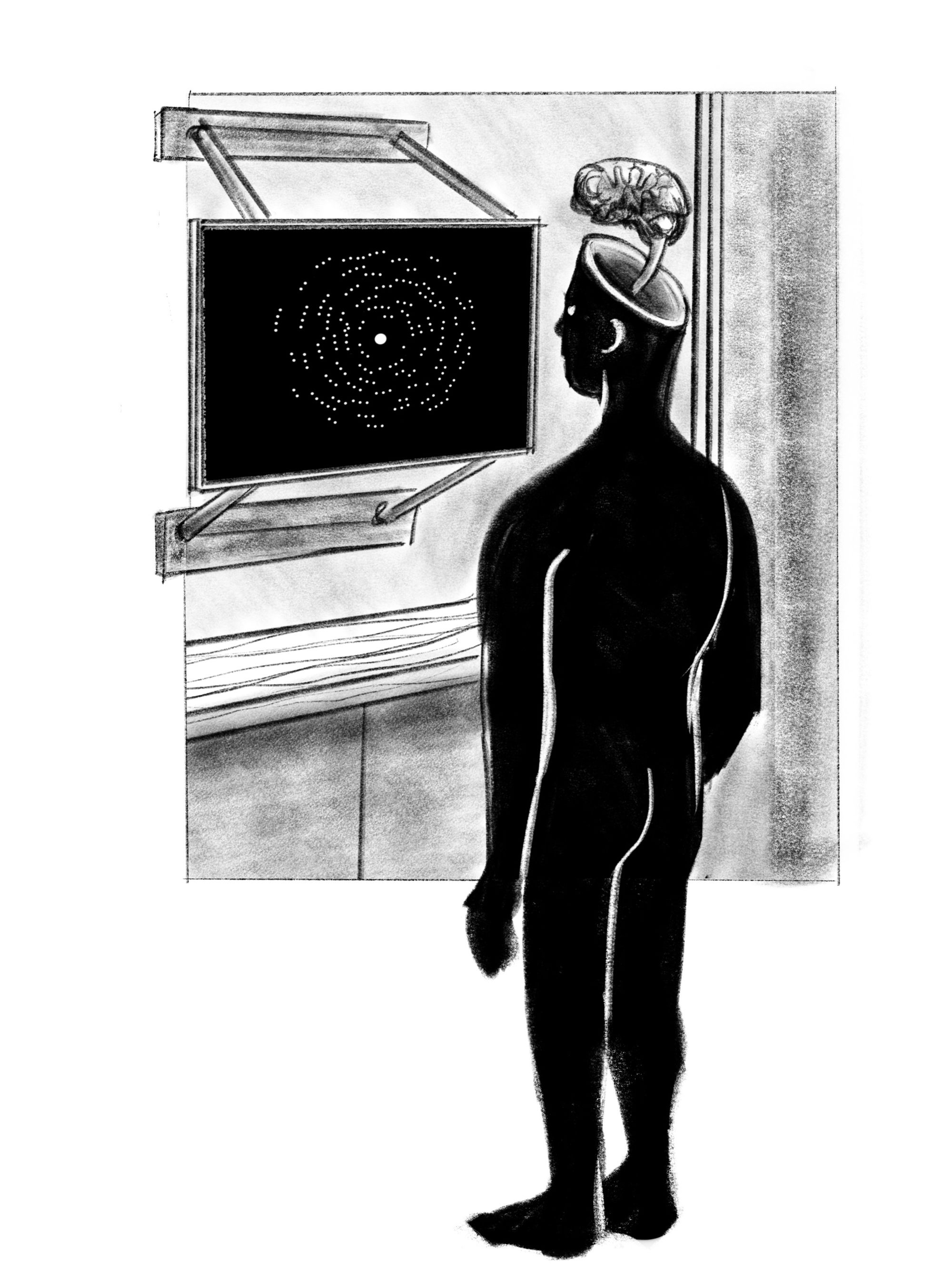
Two types of GP: Dynamic and Static
In 1969, Leon Glass created the first GPs using statistical methods to form a technique to study visual perception [4]. He copied, overlaid, and rotated a set of random dots to create a perception of pattern, and referred to it as a Glass pattern. The use of GPs thereafter became a piece within visual processing research [4].
Two main kinds of Glass patterns were formed: static and dynamic. Static Glass patterns are displayed on a 2D surface to show a pattern: they are pictures of dotted designs that do not show any defined motion. This is a single unique frame [5]. Dynamic GPs are made up of many different static GPs shown one after another, but with no net motion. Instead of a single frame, there are many. However, by showing different frames, the dynamic pattern gives off an illusion that it is moving in a certain direction [5]. A way to visualize this concept can be similar to flipping through a flip book. One page of a flip book is analogous to a static GP. The whole flip book can be referred to as a dynamic pattern. These dynamic GPs have been used to illustrate that humans perceive motion even when there is no consistency of motion [5]. Additionally, research has demonstrated that mammalian visual systems can detect dynamic patterns much better than static ones [5].
Different types of GPs can be used for researching different topics, ranging from visual perception to decision-making. Research shows that dynamic patterns are easier to process than static. This advantage may be due to how dynamic GPs can combine signals from different sources within the brain [6]. Previous studies have shown that the partner dots in GPs activate orientation detectors in the V1 (primary visual cortex) and V2 (secondary visual cortex) of the brain [7]. Furthermore, participants are unable to tell between the “implied motion” from GPs and real motion [7].
Aside from manipulating the number of frames, another way to make GPs appear differently is by modifying their coherency. Coherency is defined by how easily the pattern can be identified based on the arrangement of elements within the GP [8]. The higher the coherency, the easier it is to find the direction of a pattern within the sequence of randomly placed dots. For example, if a pattern had a 20% coherency, then the GP would have 20 paired random dots, and the rest of the dots would randomly spread the pattern without a partner dot [8]. If there was a 0% coherence condition, then none of the randomly placed dots would have their partner dot. Along the same vein, increasing the coherency would make it easier to determine the pattern. Overall, by changing the coherence, a GP can be made easier or more difficult to identify [8]. By modifying the coherence percentage, we can study visual perception and decision-making through non-human primates and humans.
Dynamic Glass Pattern and Visual Processing
There are many types of dynamic patterns, the most prevalent being translational, rotational, and circular [4]. Particularly, translational patterns have the dots aligned in a specific orientation, either right or left, at a specific distance. Circular patterns consist of rings that are arranged in a circular way. The motion perceived in circular GPs can be compared to looking at the circular ripples that form when a pebble is dropped into a pond. This circular expansion can either grow or get smaller in size and can be controlled by their coherency [4].
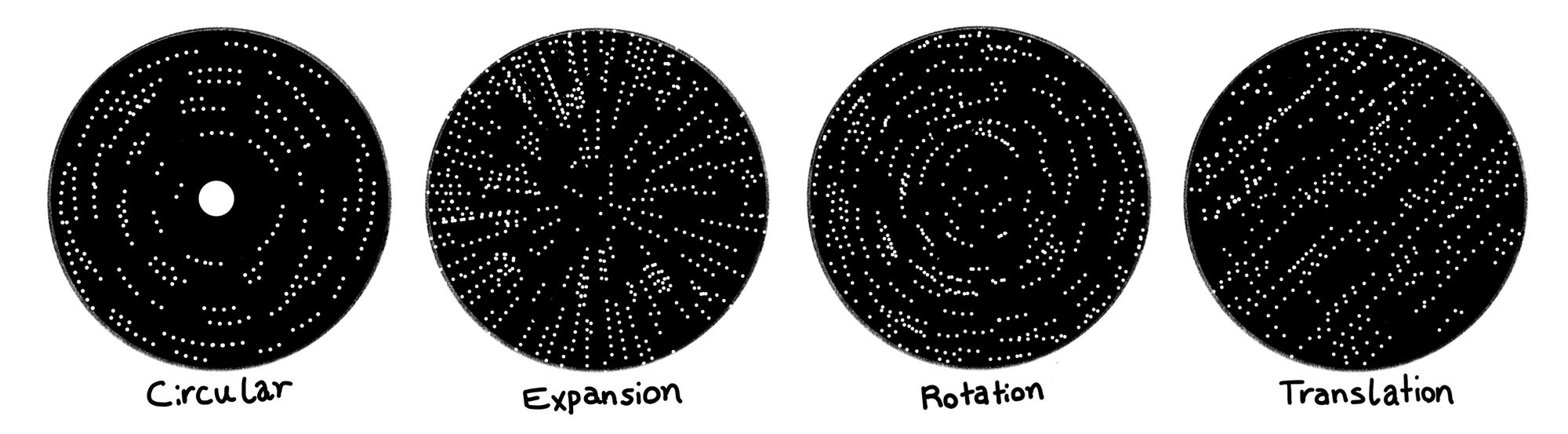
To learn more about these processes within the brain, researchers used dynamic GPs to further study the visual system and decision-making in humans [6]. The study investigated how a mammal's visual system takes in an overall view of an object and senses how that object is moving in a coordinated direction instead of perceiving motion and form separately. This experiment attempts to further investigate how humans piece random motions to perceive patterns that do not have actual movement based on the GPs. Dynamic GPs have no consistent motion, but people can perceive the random white dots as motion [6].
This study involved nine adults who looked at patterns on a screen [6]. Their heads were securely held in place with the screen at eye level. The experiment consisted of three sessions in one week to test their knowledge of patterns. During every trial, two patterns appeared on the screen. One was a random pattern with a random coherence while the other had a coherence of 0%. Finally, the participants had to decide which image had 0% coherence [6].
The experiment aimed to identify how easy it is to distinguish patterns [6]. They studied how people view patterns based on their shape and movement. It was found, once more, that dynamic patterns are easier to distinguish than static patterns. In addition, the results show that if the dynamic GPs have more frames, such as pages in a flipbook, it is easier to detect compared to a static GP. This finding shows that the adults’ heightened sensitivity is due to the increase in unique frames in the dynamic GPs [6]. It’s similar to how our brain can take a “snapshot” of our surroundings to figure out how things can move, such as when we see cars driving past us.
So what is happening in our brains to support these perceptions? There are parts of the primary visual cortex that are responsible for processing visual information. It helps us recognize shapes, lines, and patterns. Others say that the “motion streak” appearance in the GPs also stimulates the same detectors to try and piece together motion direction [6]. These findings are why we think we see motion in the dynamic GPs when they are actually just blinking dots. However, this doesn’t show why dynamic GPs are much easier to notice than static. This is where the results from the above experiments can be applied to answer why.
In conclusion, the experiment attempted to investigate whether the motion streaks from the GPs also use information from orientation detectors that detect lines or edges in the primary and secondary visual cortex [6].
Glass Pattern and Decision making
In another experiment, researchers trained non-human primates (NHPs) to make a decision based on a translational glass pattern [9]. Specifically, the researchers paid attention to the NHPs' decisions after they turned off the superior colliculus (SC). The SC is one of the main processing areas that receives significant inputs from sensory areas such as visual, auditory, and touch areas.
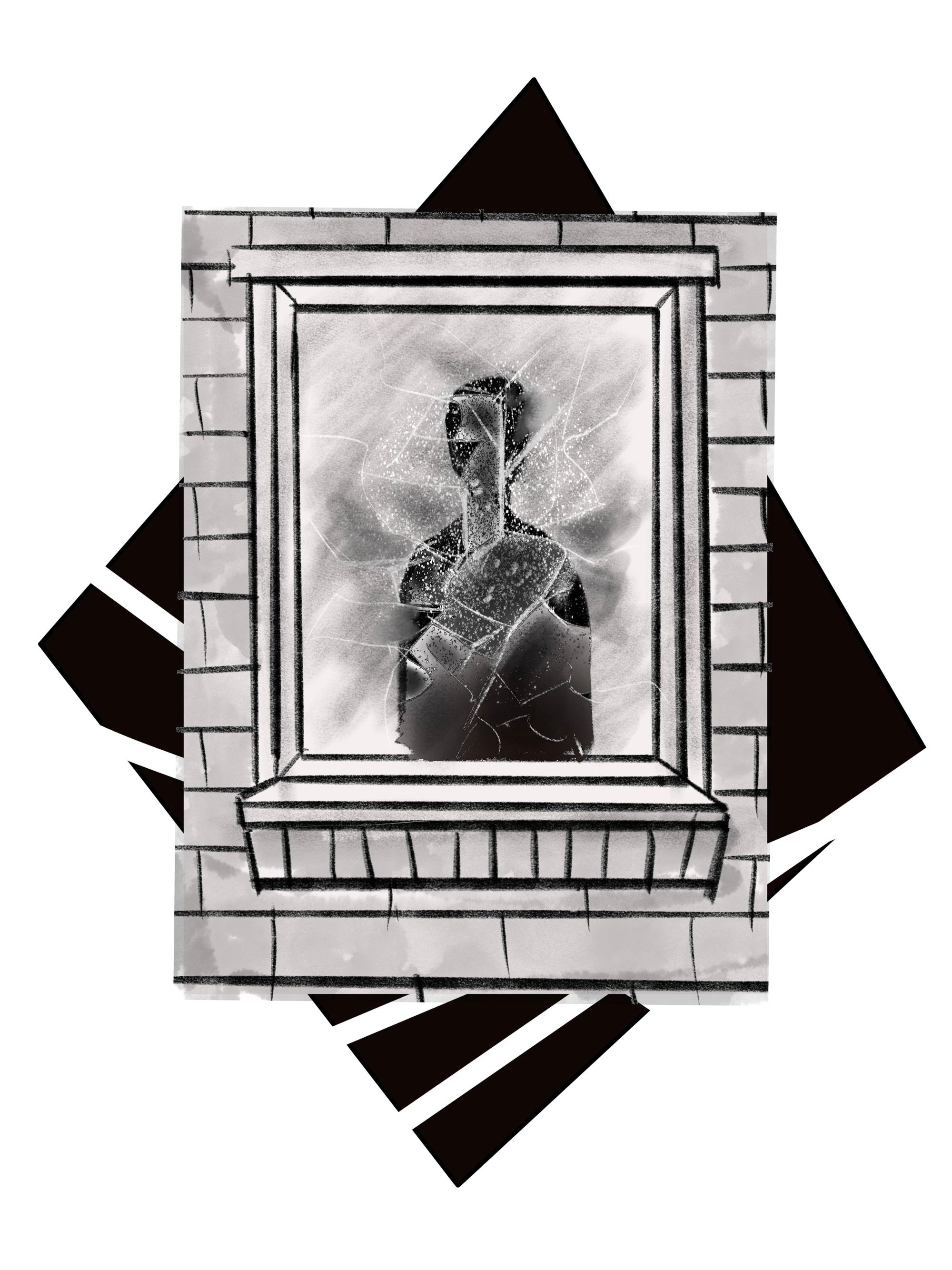
NHPs were trained to do different tasks on a portrayed screen [9]. Using specific tools, researchers were able to track the eye movements of the NHPs to determine what choice they made. In addition, they also used tools to measure their brain activity. The researchers gave the NHPs a type of task where they had to make a decision on whether the GP was shifting right or to the left. For one of the tasks, they had to wait a bit before choosing right or left. Based on their decisions, the researchers studied the SC and how it affected their decisions. When the SC was temporarily shut off, the NHPs tended to make the opposite decision. This shows that the SC is very much involved in making decisions. By looking at the activity of the neurons, they saw how much it correlated with the monkey’s choices. This experiment shows how specific regions of the brain can affect how NHPs make decisions. When the area was turned off, they noticed that the reaction time and accuracy and the monkey’s decisions were affected on one side [9].
This study helped researchers learn more and understand how the brain works [9]. Researchers could track and see what the NHPs could see because they used GPs. It helped them understand how the brain processes visual information and from that, how decisions were made. Specifically, they learned that the SC is involved in decision-making, which people thought occurred in a different region of the brain [9]. This understanding can enhance our ability to map out the neural mechanisms involved in neurological illnesses like neurodegenerative diseases and lead to the development of advanced technology for various treatments.
What’s the Purpose?
Overall, the study of Glass patterns helps us understand visual processing and decision-making, and this knowledge can be applied to improving many aspects of our lives. Research into visual processing can help us connect with many neurological conditions and vision diseases, especially neurological diseases such as Parkinson's, Alzheimer's, Autism, and traumatic brain injury. Parkinson’s disease particularly affects sensory perception in its early stages. GPs can help track the progression of the disease and the diagnosis [10]. While sensory issues are often dismissed in motor-related diseases, it is important we understand all aspects of the disease. Because of this, the current aim of studies is to model the perceptual features of Parkinson’s. In addition to neurological disorders, decision-making research can contribute to artificial intelligence (AI) performance. AI uses a decision-making system to learn patterns and rules based on a data basis. As a computer system, AI can apply what they know to specific decision-making tasks [11]. Self-driving cars, which are controlled by an AI driving system, must be able to detect their environment. To make cars fully autonomous, visual perception must be studied to be sure that cars are fully aware of their surroundings [12]. Glass patterns can help study how humans decode motion and patterns, which helps replicate human-like visual recognition for technology [12]. Therefore, studying visual perception and decision-making with Glass patterns can benefit many sectors.
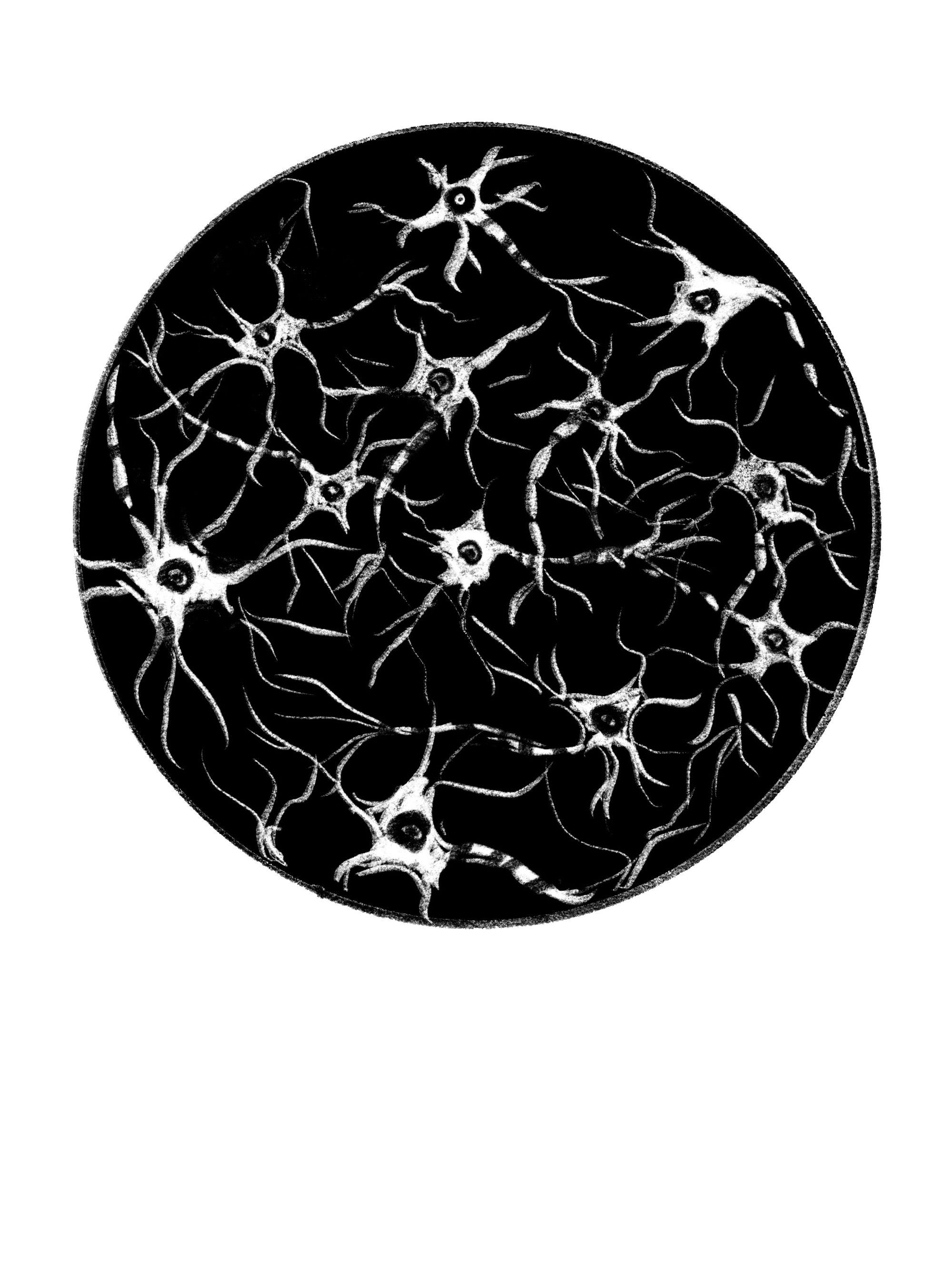
Conclusion
Glass patterns are a bit like the daily decisions we make in our everyday lives. As we make our 35,000 decisions every day, our brain is constantly interpreting patterns and movement. This is similar to the way of interpreting GPs– constantly making sense of our surroundings and making decisions based on our environment. Our journey of visual perception is like solving a puzzle, always navigating through life. As GPs continue to be used, we are advancing our understanding of how the brain works. This opens many opportunities and discoveries in science to improve disease diagnoses and technology.
Glass patterns are an important tool in scientific research. They help us explore how our complex brain works, specifically how it processes shapes and movement. They are necessary as they help us uncover how our brains make sense of our visual experiences in our world, which can provide advances in learning more about neurodegenerative diseases, such as Parkinson's. They provide hope for better diagnostics and even treatments, as they allow researchers to dive into visual perception and advancing fields like AI. Next time you spot an illusion, rest assured that it is not just a trick, you’re actually unlocking the meaning of the secret in your remarkable, intelligent brain.
References
- Graff, F. (2022, August 10). How many decisions do we make in one day? PBS North Carolina. https://www.pbsnc.org/blogs/science/how-many-decisions-do-we-make-in-one-day/
- Nankoo, J.F, Madan, C.R., Spetch, M.L. (2012). Perception of dynamic glass patterns. Vision Research, 72, 55-62. https://doi.org/10.1016/j.visres.2012.09.008.
- Smith, M. A., Bair, W., & Movshon, J. A. (2002). Signals in macaque striate cortical neurons that support the perception of glass patterns. The Journal of neuroscience: the official journal of the Society for Neuroscience, 22(18), 8334–8345. https://doi.org/10.1523/JNEUROSCI.22-18-08334.2002
- Glass, L. & Smith, M.A. (2011). Glass patterns. Scholarpedia, 6(8). http://www.scholarpedia.org/w/index.php?title=Glass_patterns&action=cite&rev=137324
- Donato, R., Pavan, A., Almeida, J., Nucci, M., & Campana, G. (2021). Temporal characteristics of global form perception in translational and circular Glass patterns. Vision research, 187, 102–109. https://doi.org/10.1016/j.visres.2021.06.003
- Nankoo J.F., Madan C.R., Spetch M.L., Wylie D.R. (2015). Temporal summation of global form signals in dynamic Glass patterns. Vision Research, 107, 30-35. https://doi.org/10.1016/j.visres.2014.10.033.
- Ross, J., Badcock, D. R., & Hayes, A. (2000). Coherent global motion in the absence of coherent velocity signals. Current biology: CB, 10(11), 679–682. https://doi.org/10.1016/s0960-9822(00)00524-8
- Qadri, M. A., & Cook, R. G. (2015). The perception of Glass patterns by starlings (Sturnus vulgaris). Psychonomic bulletin & review, 22(3), 687–693. https://doi.org/10.3758/s13423-014-0709-z
- Jun, E. J., Bautista, A. R., Nunez, M. D., Allen, D. C., Tak, J. H., Alvarez, E., & Basso, M. A. (2021). Causal role for the primate superior colliculus in the computation of evidence for perceptual decisions. Nature neuroscience, 24(8), 1121–1131. https://doi.org/10.1038/s41593-021-00878-6
- Bernardinis, M., Atashzar, S. F., Jog, M. S., & Patel, R. V. (2023). Visual velocity perception dysfunction in Parkinson's disease. Behavioural brain research, 452, 114490. https://doi.org/10.1016/j.bbr.2023.114490
- Samoili, S., Lopez C.M., Gomez G.E., De Prato, G., Martinez-Plumed, F. and Delipetrev, B. (2020). AI WATCH. Defining Artificial Intelligence. Publications Office of the European Union. https://doi:10.2760/382730
- Häne C, Heng L, Lee GH, Fraundorfer F, Furgale P, Sattler T, Pollefeys M. (2017). 3D visual perception for self-driving cars using a multi-camera system: Calibration, mapping, localization, and obstacle detection. Image and Vision Computing, 68, 14-27. https://doi.org/10.1016/j.imavis.2017.07.003.